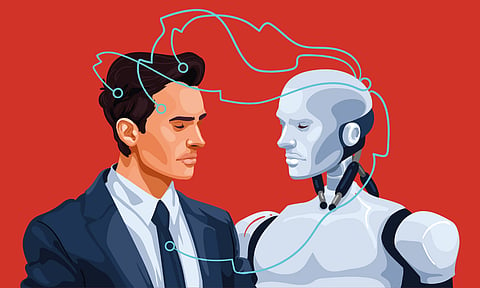
Hyderabad: AI has become a hot button issue nowadays, sparking widespread discussions and concerns about its potential to replace jobs. As it permeates various aspects of our lives, questions arise about how we should view AI – as a source of fear or a force set to dominate our existence.
Beyond its mere existence, there are pressing concerns about the potential harm Artificial Intelligence systems may cause. Questions about accountability for damages, ownership of intellectual property, the nature of data fed into AI systems, and their susceptibility to societal inequalities need addressing.
Unknown avenues
Some questions arise in light of AI’s impact on society: Is AI indifferent to inequalities in our culture? Can entirely objective data be procured for AI systems to utilise? How might Artificial Intelligence be employed simultaneously in multiple legal systems with differing moral standards and legal frameworks? What systems and procedures can effectively rectify the issues related to the application of AI? In the context of Artificial Intelligence, how should the promotion of innovation and the mitigation of risks be balanced?
Navigating these questions is indeed challenging, given the continuous evolution of AI. Contextualising these discussions is a starting point for finding answers.
Ethical quandary
There are a few pillars that AI governance and its dependant structures need, to ensure that AI remains ethical. AI doesn’t exist in isolation; considerations about Big Data, Platforms, and power dynamics are integral to the conversation.
The process is as vital as the product itself, emphasising the need to focus on the processes shaping AI-based products. Inclusivity in product design is a crucial consideration that needs to be accounted for in the development of a product. An overarching question is always raised regarding the individuals building these AI systems and whether they are taking adequate measures to prevent the reflection of societal inequalities in these machine-based systems.
Subjecting AI to Turing test
Thought experiments, such as the Chinese Room argument, shed light on society’s impact on AI. This experiment challenges the notion that a machine, despite its apparent intelligence, lacks true understanding and consciousness. This experiment also emphasises the fact that thinking constitutes understanding, and if a machine is not thinking, it cannot be said to be understanding in the way, a human being is. This test is also known as a Turing test.
The Chinese Room experiment goes as follows: Suppose a person, X, is confined to a room with no understanding of the Chinese language. Armed with only paper, pencil, and a guidebook instructing how to respond to specific queries. The room is full of Chinese-speaking people. Now person X receives questions in Chinese from these individuals. Despite lacking genuine comprehension of the language, person X consults the guidebook and formulates responses without true understanding.
The underlying argument presented here is that a computer or machine is just a resemblance of person X – that it does nothing more than adhere to predefined rules in an instruction guide. The machine lacks true understanding of the questions posed to it or its responses, thus it cannot be said or deemed to be ‘thinking.’
The machine, devoid of genuine understanding or intentionality, cannot be ascribed the attribute of ‘thinking’ and, consequently, does not possess a ‘mind’ in the conventional sense. Therefore, a machine cannot be considered intelligent.
The knowledge argument begins with the proposition that a person can acquire complete knowledge of all the physical and functional aspects associated with a particular kind of phenomenal experience without truly grasping what it is like to undergo that experience.
Lived experiences Vs derived knowledge
Let’s consider another example:
A woman named Mary has lived her life entirely within the confines of a black-and-white room, devoid of exposure to colours. Yet, she dedicates herself to mastering every conceivable physical aspect associated with colour perception. In short, Mary learns everything physical about seeing red. No physically described facts escape her. Despite this exhaustive knowledge, a crucial dimension seems to elude Mary – she lacks an understanding of what it’s like to actually see red, hereafter as WIL (What It’s Like).
Upon her release from the monochromatic room, Mary learns WIL and is finally introduced to the experiential aspect of seeing red; she witnesses roses and ripe tomatoes. She observes the vividness she had previously been devoid of, and now her newfound experiences enrich her understanding of the mysterious colour they call ‘red.’
Now Mary knows what red is like; ‘Red like that,’ she says while looking up at a cardinal in the park. Mary was earlier ignorant of the nature of seeing these colourful phenomena and unaware of the perceptions of the enigmatic colour known as ‘red,’ despite knowing all the physical aspects of it. Mary gained a profound insight after her release. The crux of the knowledge argument is that despite Mary’s exhaustive knowledge of the physical facts related to colours, such knowledge falls short of providing a complete understanding of what it’s like. Knowledge of physical facts might allow us to arrive at the knowledge of what it’s like through imagination.
Removing biases and discrimination from AI
These experiments underscore the importance of human experiences and understanding in making ethical decisions. Technology comes with its biases prevalent in society and so does AI. The society that we live in today is fairly complex and surrounded by biases that inadvertently become ingrained in the information fed to AI machines during decision-making processes.
For example, in 2014, Amazon developed a recruiting tool to identify potential software engineers it might want to hire. However, the system swiftly began discriminating against women, prompting the company to abandon it in 2017. This scenario highlights a fundamental problem at the core of AI; that even a thoughtfully designed algorithm must make decisions based on inputs from a flawed, imperfect, unpredictable, idiosyncratic real world. ProPublica analysed a commercially developed system that predicts the likelihood that criminals will re-offend, created to aid judges in making better sentencing decisions. It was revealed to have biases against individuals of Black ethnicity.
These thought experiments collectively emphasise the imperative of being human to experience certain phenomena and make choices. A profound understanding of certain concepts is necessary to make ethically sound decisions. The overarching question, then, is what is understanding and what is its truest and complete form?
The Chinese Room experiment envisions AI as a mechanistic entity that merely responds to data mechanically without having a genuine understanding of it. Understanding socio-political contexts and the inherent biases of society is something that cannot be fed into AI machines. This needs to be accounted for when thinking of AI.